In the first release of the 5G standard, the Network Data Analytics Function (NWDAF) was ratified as part of the new network architecture. As defined in several 3GPP specs (TS 23.288, TS 23.501, TS 23.502, TS 29.510, TS 29.520, and TS 33.501), the NWDAF incorporates standard web-based interfaces from the service-based architecture to collect data by subscriptions and notifications from other Network Functions (NF), which we covered in our first NWDAF blog. Since the first release, this core network function has expanded significantly from its initial use case of network slice load monitoring to serve the Policy Control Function (PCF) and the Network Slice Selection Function (NSSF).
With Release 16 and Release 17 (expected to be finalized in June 2022), the standard includes multiple data producers and consumers (from the Access and Mobility Management Function (AMF) to the Unified Data Management NF (UDM), adding about sixteen new use cases, ranging from NF load analytics to session management congestion control. With each new release, the NWDAF gains more and more capabilities that show how the evolution of this core function could become a critical function in the 5G core and the central hub for automation.
Why automate?
Automation is defined as a wide range of technologies that reduce human intervention. Like at the beginning of the aviation industry, it was clear that automating certain functions were necessary to make air travel safer (studies show that most crashes were caused by human error) and to make bigger and better planes. Automation does not replace people but alleviates workloads and ensures flight crews can focus on more critical tasks—the same for the telecom industry as we fly towards a 5G future.
The initial use case for the NWDAF of network slicing is an excellent example of why automation will be essential in 5G. However, we have covered this in a previous blog. One of the services expected to be delivered using network slicing is the Internet of Things (IoT) which is a more interesting use case to examine. It also emphasizes why isolation within the components of a slice is essential to prevent vulnerabilities or malicious attacks.
5G supports up to 100 times more devices per square kilometer than 4G, so the term Massive IoT (mIoT) has been coined to describe future IoT. With the real possibility of billions of new devices connecting to the network, operators will need to carefully monitor and analyze IoT services for SLAs and security threats. Yet, it presents a significant challenge to operators, especially when considering that an operator’s network could be connected to millions of devices ranging from critical smart factory sensors to mobile-connected drones. This complexity scale will not be manageable using manual processes, and automation will be essential.
Enter the NWDAF that will enable vital mIoT anomaly detection—automatically detecting anomalies that indicate network and security issues associated with IoT devices. IoT traffic is monitored at multiple levels – per UE, group, and device type. In addition, traffic is monitored according to communication intervals, traffic volumes UL and DL, traffic source/destination IP address/port, and the geo-location of the UE (cell/sector).
The AI/ML-based anomaly detection and clustering analysis are applied to the IoT CDRs to automatically detect and classify anomalies, including network and potential security issues. For example, an IoT device transmitting at an unexpected time, NOT sending at an expected time, communicating to an abnormal destination, transmitting, or receiving abnormally high traffic volumes, and an IoT device that has moved to an abnormal location (cell/sector). When this behavior is detected, alerts to 3rd party systems are triggered, e.g., to 3rd party Network Attack Prevention and Mitigation solutions. In addition, corrective action is automatically initiated, e.g., barring suspicious IoT devices from the network.
Analytics for automated assurance
As well as automation and closed-loop operations, the NWDAF is also a key enabler for automated service assurance in 5G. For example, the NWDAF can automatically monitor many physical and logical objects 24/7/365, detecting anomalies, performing predictions, running automated root-cause analysis, and triggering corrective actions for a wide range of network issues. These analytics automatically enable the resolution of network issues that are already occurring in real-time and offer prediction and prevention of network issues before they occur.
The cornerstone for autonomous networks
The NWDAF combines Artificial Intelligence (AI), Machine Learning (ML), and centralized analytics. It is helping address complex challenges like monitoring millions of IoT devices, performing intelligent slice selection, and laying down the cornerstone to building autonomous networks for network slicing and other network management tasks. Ensuring that operators can manage the complexity of 5G using a standardized analytics system that allows multi-vendor interworking and forms a critical launchpad for an operator to automate network functions. It’s also crucial that the NWDAF is vendor-agnostic and cloud-agnostic. It serves as an independent auditor of the 5G core to provide impartial analytics of the service quality and network performance. In short, the NWDAF can become a system like an autopilot that allows the lifecycle of the network to be controlled autonomously.
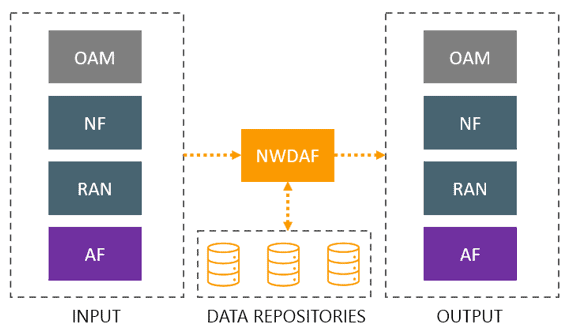
The challenges
Although some advanced NWDAFs have the option to collect and process data via probes and tap the network interfaces, for others that only reply on network events, Network Equipment Providers (NEP) and other vendors need to expose their network events to the NWDAF. However, as this data exposure is costly and increases signaling traffic generated by the different network functions, some NEPs and vendors may be hesitant to do this. So, telecom operators may need to push for this in their 5G SA network planning processes.
The one DAF to rule them all?
Although the NWDAF is the most well-known Data Analytics Function (DAF), the 5G-PPP Architecture Working Group View on 5G Architecture Version 4.0 and the End-to-End Data Analytics Framework for 5G Architecture both describe multiple data analytics functions (DAFs); core network domain (NWDAF), big data and management and orchestration (big data/MDAF), application function level (AFDAF), user equipment/RAN-DAF and data network (DN-DAF). With multiple DAFs, some operators may deploy different DAFs across the network. There are pros and cons to having separate DAFs for the Core, RAN, etc., vs. one centralized DAF to rule them all (e.g., the NWDAF) and serve all domains.
To probe or not to probe
Insights from cloud-native, probe-based assurance are an essential source of data for service assurance, subscriber and network troubleshooting, and QoE monitoring. This will remain even when NWDAF is deployed, as we covered in a previous blog post. In fact, for 5G, this data becomes even more vital with all the new technologies such as the Open RAN, RAN using different spectrum waves, 5G core, etc. In all technology transitions, probe-based data provides the real-time subscriber analytics and correlated user sessions needed to iron out kinks in the network and optimize service quality that network event data cannot offer. Often, regulators expect telecom operators to store raw network traffic for a certain period, which is also a task provided by probes.
Other examples of NWDAF automation
Multi-slice resource management
Managing a multitude of network slices with diverse performance requirements, business requirements, and slice-specific protocol configurations will be challenging. Management of these various slices will require a unified multi-slice resource management system coupled with fully integrated performance monitoring to fulfill SLAs of the network slices while ensuring resource isolation between slices. Also, slices with mission-critical services impose further constraints on the SLA fulfillment. Here the NWDAF will provide SLA monitoring and insights on SLA breaches that can be used to adjust performance thresholds to prevent this. In addition, predictive analytics will provide insights into slice load variations over time and be used for resource and capacity allocations and cell range extension parameters.
Signaling storm prevention
The NWDAF detects a signaling storm by monitoring signaling traffic rates in the Control Plane NFs such as AMFs, SMFs, etc. The built-in AI/ML is applied to the signaling traffic rate data to automatically detect and isolate the root cause and UEs and establish which gNodeBs generate the signaling storm. As the NWDAF sees a storm is starting and before the storm severely affects the NFs, the NWDAF triggers corrective actions to prevent the storm, such as temporarily barring UEs or reconnecting UEs to other AMFs.
Energy Efficiency Improvements
Using the NWDAF, operators can transition to a more sustainable 5G ecosystem with intelligent power optimization and energy-saving techniques to dynamically manage their resources. For example, turning off under-loaded cells and reassigning subscribers to another cell will improve network energy efficiency. At the same time, predictive analytics will be provided by the NWDAF to determine the expected load of a cell or cell group. That way, ensuring an optimal trade-off is made between energy efficiency gains vs. user performance reduction due to the higher burden of the active cells that can be reached by factoring in the load history and activities in neighboring cells.
Conclusion
To summarize, the NWDAF is the conductor interpreting the score, setting the tempo, and ensuring correct entries by all its members. That effortlessly manages and guides all the parts of its orchestra to deliver a magnificent tune and ensure customer satisfaction.
However, to deploy an NWDAF, the following essential ingredients are needed. The first is that the NWDAF is cloud-agnostic, vendor-agnostic, and visibility-agnostic to collect and analyze data from multiple domains and sources openly and impartially. Built-in AI/ML that is telecom-domain specific. Access to a rich data set of information such as network packets, network events, and event subscriptions to guarantee comprehensive coverage of the 5G network. Finally, end-to-end analysis is critical, spanning from the RAN to the network core.
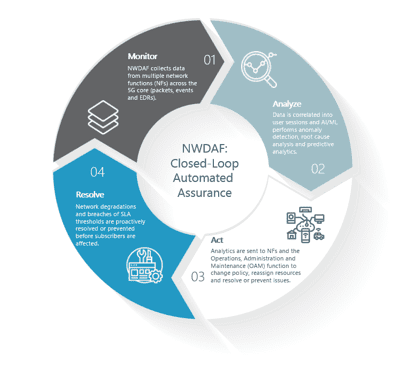
Once these are in place, the operator will have a centralized analytics function that feeds the collected data into AI/ML models for automated closed-loop operations and automated assurance for automation and predictive analytics. Utilizing the power of AI and ML to become a critical function in the 5G core and the cornerstone for autonomous networks and helping deliver zero-touch network operations that minimize operational costs and ensure lean and efficient network operations.
For more information, visit https://radcom.com/products/products-radcom-nwdaf/.
This blog was published as part of Light Reading’s Partner Perspective series.
The article is subject to RADCOM’s disclaimers regarding Forward-looking statements and general information under the links below: